Doctoral Candidate – no. 2
Technische Universiteit Delft – TU-DELFT
Application of Machine Learning to Wind Resource Assessment
Scope and Objectives
Increasing the accuracy and reducing the uncertainty of long-term wind resource estimates when planning wind farms has the potential to increase P90 values, reducing the cost of capital and thus investment costs.
Only in the last few years, has attention been focussed on the application of machine learning (ML) to wind resource assessment. The traditional approach has focussed on the use of numerical models and relatively simple statistical techniques such as Measure-Correlate-Predict (MCP).
The availability of large amounts of meteorological and digitised terrain data from terrestrial and remote sensing equipment (e.g., satellites, lidar) as well as the ever-increasing resolution of reanalysis datasets provide a rich source of information for site specific resource assessment.
Indeed, the proliferation of wind farms can provide an additional source of data, though access to such data may be commercially restricted.
The application of machine learning to unlock the potential of these data is at an early stage. The researcher will investigate how ML can be used to produce more accurate resource assessments as an alternative to physical modelling and as a supplement considering onshore and offshore applications.
Expected Results
The fellow will be trained in the application of ML techniques to the prediction of long-term site specific wind resource to improve accuracy and reduce uncertainty in energy yield estimates.
Verification and validation of the ML predicted resource estimates will be made using real wind farm site data benchmarking against existing techniques in order to asses improvements in accuracy.
Planned secondments
Two secondment periods (3 months) are foreseen for collaboration on reducing uncertainties in energy yield estimates, DTU (Dr. Ju Feng, M24-M26) and to develop and test ML techniques for resource assessment, DNV (Lars Landberg, M35-M37).
Develop your career
Don’t miss this opportunity to pursue your passion and make a meaningful contribution
Doctoral Candidate
Name Candidate
-
Supervisor
Prof. Simon Watson
- s.j.watson@tudelft.nl
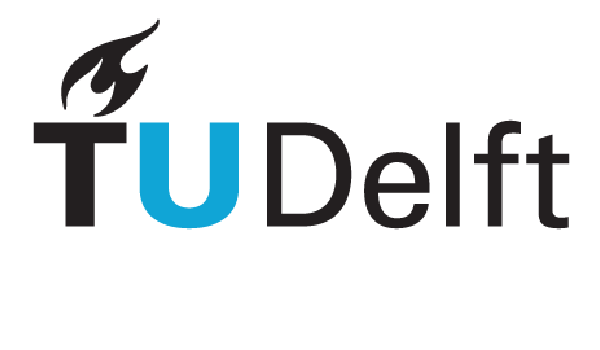